Energy storage technology, as an important supporting technology for the new energy industry, is commonly used for peak shaving and valley filling of grid power, overcoming the intermittency of renewable energy generation, improving power quality, and providing power sources for transportation vehicles such as electric vehicles, light rail trains, and ships. With the development of energy storage technology, lithium-ion batteries (LIBs) have become the most widely used energy storage devices in the new energy industry due to their high energy density, good power performance, extremely low self discharge rate, and low cost. However, energy storage systems composed solely of LIBs still have many shortcomings, such as severe performance degradation and rapid life decay when used at low temperatures, high rate pulse discharge, and large fluctuations in power throughput requirements, which can also lead to rapid aging of LIB energy storage systems; In addition, due to the low power performance of LIB, it is necessary to stack too many battery cells in application scenarios with high power demand, low energy demand, or long service life requirements for energy storage systems, which leads to excessive system volume and weight, and high costs for battery procurement, operation, and management.
Therefore, how to improve the working conditions of LIB and slow down its capacity degradation has become the core issue of energy storage technology research. Combining two or more energy storage systems into a hybrid energy storage system can leverage strengths and avoid weaknesses, effectively solving the problems of low temperature, high rate pulse discharge, and power fluctuations affecting the lifespan of LIB systems; The power and energy devices in hybrid energy storage system can be flexibly configured according to application requirements, avoiding excessive stacking of battery cells and reducing the cost of purchasing, operating, and managing energy storage systems.
The performance parameters of current mainstream energy storage systems are shown in Table 1, where supercapacitors (SC) are similar to LIBs in terms of volume, cost, working mode, calendar life, and other application conditions. However, they can complement LIBs in terms of electrical performance such as power density, energy density, self discharge rate, and cycle life, hybrid energy storage system composed of LIB and SC has been widely used as a power source or power buffer system for various types of electric vehicles, as well as a supporting energy storage system for power grids, wind farms, and photovoltaic power stations; At present, research on hybrid energy storage system in the academic community is mostly based on hybrid energy storage system composed of LIB and SC.
Energy storage type | Power density/(W/kg) | Energy density/(Wh/kg) | Daily self discharge rate/% | Response time Efficiency/% | Calendar | Calendar lifespan/year | Cycle life/time |
Compressed air | \ | 30-60 | 0 | seconds to minutes | 40-70 | 20-40 | \ |
Flywheel | 400-1 600 | 5-130 | 20-100 | milliseconds to seconds | 80-90 | 15-20 | 104~107 |
Supercapacitor | 3 000-50 000 | 5-20 | 2-40 | milliseconds | 85-98 | 5-12 | 105~106 |
Lead-acid battery | 20-200 | 30-50 | 0.1-1 | milliseconds | 78-85 | 5-10 | 500-2 000 |
Lithium ion batteries | 250-450 | 130-250 | 0.1-0.3 | milliseconds | 80-95 | 5-10 | 1 000-3 000 |
Sodium ion battery | 100-120 | 100-150 | 0.1-0.2 | milliseconds | 80-90 | 1-5 | 1 000-1 200 |
Liquid flow battery | \ | 30-50 | 0-1 | milliseconds to seconds | 70-85 | 10-20 | >104 |
Fuel cell | 3 000-5 400 (W/L) | 500-3 000 | 0.5-2 | seconds to minutes | 20-66 | 5-30 | 103~104 |
Although lithium-ion battery/supercapacitor (LIB/SC) hybrid energy storage systems have significant advantages compared to pure LIB energy storage systems, to achieve complementary advantages between LIB and SC, it is necessary to scientifically and reasonably allocate the real-time power of the load between the two, which poses a significant challenge to the energy management strategy design of LIB/SC hybrid energy storage systems. Therefore, starting from the topology structure of LIB/SC hybrid energy storage system, this article briefly introduces the energy management system (EMS) framework of LIB/SC hybrid energy storage system and the underlying control process of power allocation. Then, the focus is on classifying and comparing the energy management methods of LIB/SC hybrid energy storage system. Finally, the characteristics of existing energy management methods are summarized, And the development trend and next research directions of energy management in LIB/SC hybrid energy storage systems were prospected.
1. Overview of energy management technology for hybrid energy storage systems
1.1 Overview of Hybrid Energy Storage System Topology Structure
The selection of hybrid energy storage system topology determines whether it can be controlled for power allocation through hybrid energy storage EMS. According to whether the power distribution between subsystems is controlled, the existing LIB/SC hybrid energy storage system topology can be divided into two categories: controlled topology and uncontrolled topology.
In a controlled hybrid energy storage system, the hybrid energy storage EMS achieves power distribution between subsystems by controlling the bidirectional DC/DC converter. According to the number and connection method of bidirectional DC/DC converters, the controlled hybrid energy storage system topology can be divided into two types: fully active topology and semi active topology, as shown in Figure 1 (a) and Figures 1 (b) and (c).
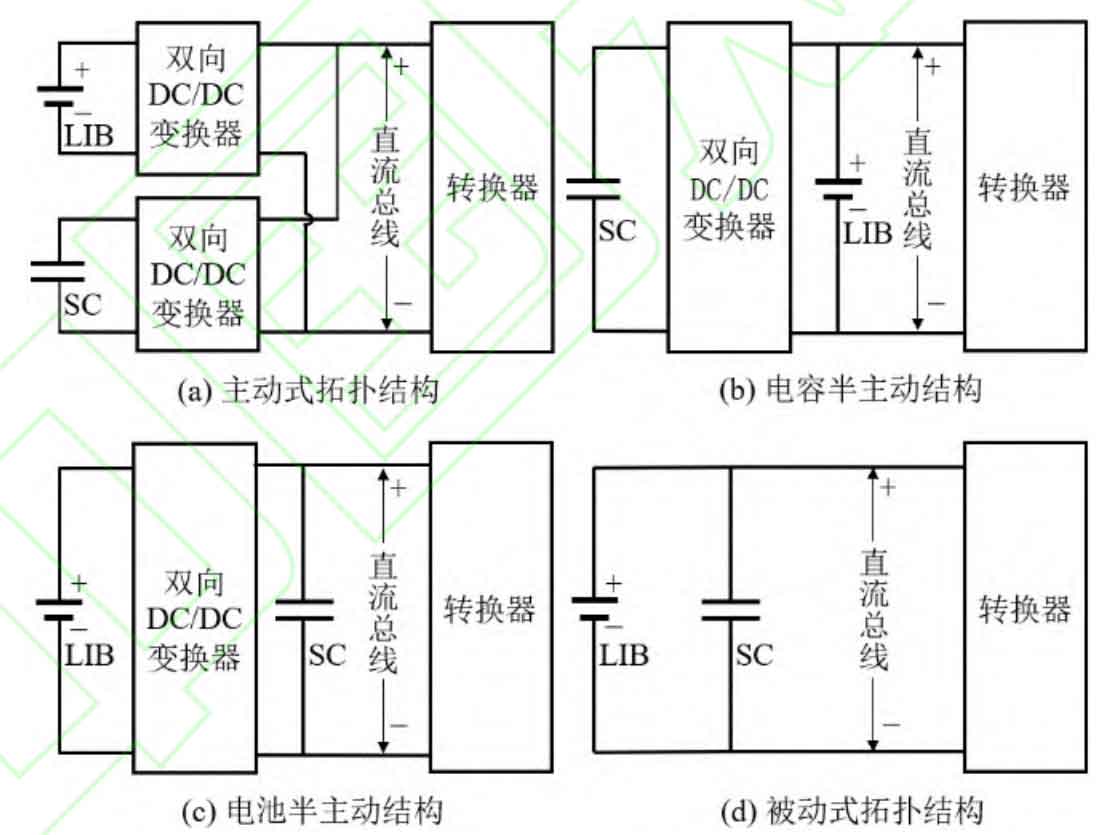
Figure 1 (a) shows the topology of a fully active hybrid energy storage system. In this topology, LIB and SC subsystems are connected to the DC bus through bidirectional DC/DC converters, respectively; At this point, the output power and bus voltage of each subsystem are controllable, suitable for application scenarios with high requirements for bus voltage stability.
The main function of a controlled hybrid energy storage system topology is to achieve controlled current distribution between the LIB subsystem and the SC subsystem. When the power demand of the load is constant, the hybrid energy storage system with a semi-active structure only controls the output power of the subsystems connected to it through a bidirectional DC/DC converter, and the other power demands are supplemented by another subsystem, which can also achieve controlled power distribution; But at this point, the bus voltage is no longer controllable. Figures 1 (b) and 1 (c) show two different configurations of semi-active hybrid energy storage system. When the SC subsystem is connected to the bus through a bidirectional DC/DC converter, and the LIB subsystem is directly connected to the bus, it is called a capacitive semi-active structure, and vice versa, it is called a battery semi-active structure; Semi active structures, especially capacitive semi active structures, are currently the most commonly used hybrid energy storage system topology structures.
In addition, based on the various types of controlled hybrid energy storage system topologies mentioned above, some scholars have added controlled switches, adjusted connection methods, and switched to unidirectional DC/DC methods according to the actual operating characteristics of hybrid energy storage system applications, in order to achieve targeted reduction of converter weight and improvement of energy utilization efficiency based on application scenarios. However, its core function is still to achieve controlled allocation of subsystem input and output power.
The uncontrolled hybrid energy storage system does not use DC/DC converters, and the LIB subsystem and SC subsystem are directly connected to the DC bus. This type of topology is called passive topology, which has the advantages of simple structure and low cost. Figure 1 (d) shows a common passive hybrid energy storage system topology structure. Based on this typical passive structure, a few scholars have connected inductors or diodes in series in the battery branch according to application requirements to reduce current ripple or control current direction. Overall, all types of passive hybrid energy storage system cannot achieve controlled distribution of subsystem power, and the power output ratio of each subsystem is determined by its own internal resistance.
HESS topology | Output current of battery subsystem | Output voltage of battery subsystem | Output current of capacitor subsystem | Output voltage of capacitor subsystem | Bus voltage |
Controlled active topology | Controllable | Controllable | Controllable | Controllable | Controllable |
Semi active topology battery semi active | Controllable | Controllable | Uncontrollable | Uncontrollable | Uncontrollable |
Semi active topology capacitor semi active | Uncontrollable | Uncontrollable | Controllable | Controllable | Uncontrollable |
Uncontrolled passive topology | Uncontrollable | Uncontrollable | Uncontrollable | Uncontrollable | Uncontrollable |
The core of hybrid energy storage system energy management is to achieve scientific and reasonable allocation of load demand power between LIB and SC subsystems. For hybrid energy storage system topology, only controlled topology can achieve controlled power allocation between subsystems. Therefore, the energy management method described in this article is applicable to controlled hybrid energy storage system such as active and semi-active ones, while uncontrolled (passive) hybrid energy storage system cannot perform energy management. The summary of various hybrid energy storage system controlled parameters is shown in Table 2.
1.2 Overview of Hybrid Energy Storage System Topology Structure
2.2.1 Functional Architecture of Hybrid Energy Storage Energy Management System: Hybrid Energy Storage EMS is the key to ensuring the economic and stable operation of hybrid energy storage system and fully leveraging the performance advantages of LIB and SC. Its main function is to make decisions and control the input and output power of each subsystem based on the collection, processing, and analysis of temperature, bus, and voltage and current information of subsystems, in order to meet the real-time power demand of the load, To extend the lifespan of hybrid energy storage system, improve energy utilization, and achieve stable, efficient, and economical operation of the system. In addition, hybrid energy storage EMS also needs to have the functions of traditional battery management system (BMS) in measurement, evaluation, balancing, and protection.
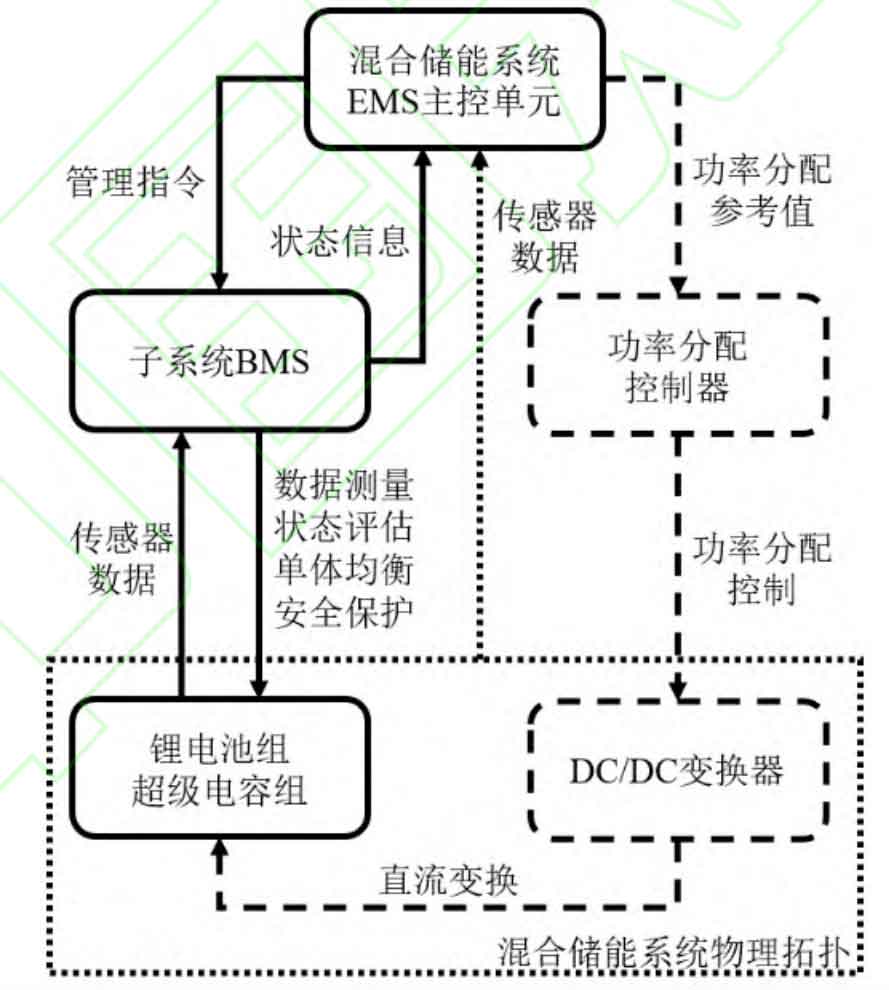
The functional architecture of the hybrid energy storage EMS is shown in Figure 2. The hybrid energy storage EMS main control unit mainly has three functions:
1) Collect voltage, current, and temperature data from the bus, LIB subsystem, and SC subsystem through various sensors.
2) Communicate with the subsystem BMS to obtain state of charge (SOC), state of health (SOH) and other status information of the LIB subsystem and SC subsystem, and issue management instructions. Through the subsystem BMS, complete BMS functions such as voltage/current/temperature data measurement, SOC and SOH estimation, single SOC balance and redistribution, thermal management and overvoltage/overcurrent/temperature protection for individual units within the subsystem.
3) For hybrid energy storage system with controllable subsystem power output, the hybrid energy storage EMS main control unit needs to combine the received sensor data and subsystems
Based on the real-time power demand of the load, decisions are made on the input and output power of each subsystem based on information such as state data, and
The obtained power allocation reference value is communicated to the controller to achieve power allocation control; For hybrid energy storage system with controllable bus voltage, EMS master control
The unit also needs to control the bus voltage, which is similar to the power distribution control mentioned earlier and will not be repeated here.
It can be seen that for hybrid energy storage system with controllable subsystem power output, the main function of EMS is to collect necessary data and control power allocation to achieve efficient, economical, and long-life operation of hybrid energy storage system; The traditional BMS functions of measurement, evaluation, balancing, and protection are completed by the subordinate subsystem BMS. When the power output of the subsystem is uncontrollable, the functional architecture of the hybrid energy storage EMS no longer includes the power allocation control part (i.e. the short dashed box and arrow part in Figure 2). At this time, the hybrid energy storage EMS is actually equivalent to a modular BMS. Due to the limitations of the topic, this article will not discuss the relevant management methods for this type of BMS.
2.2.2 Hybrid Energy Storage Energy Management System Power Allocation Control The hybrid energy storage EMS main control unit relies on the energy management algorithm running on it to provide power allocation reference values to the lower level controller. Then, the lower level controller controls the DC/DC converter in the controlled hybrid energy storage system topology to achieve power allocation between subsystems. For semi-active hybrid energy storage system, the low-level control only involves power allocation control between subsystems; The active hybrid energy storage system also needs to control the bus voltage. Due to the fact that the underlying control problem of semi-active topology is a subset of the underlying control problem of active topology, the following will only provide an overview of active hybrid energy storage system in terms of underlying control.
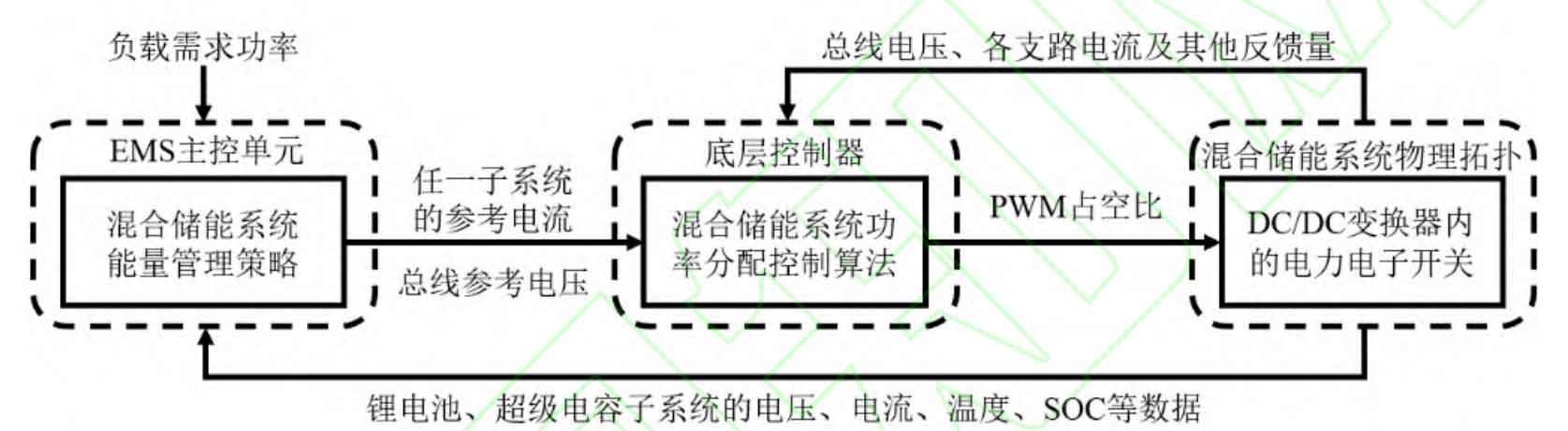
Figure 3 shows the general process of active hybrid energy storage system power allocation control. Among them, the hybrid energy storage system energy management algorithm runs in the hybrid energy storage EMS main control unit, receiving real-time load demand power and voltage, current, temperature, SOC and other data of LIB and SC subsystems as inputs to the energy management algorithm. Based on the aforementioned input data, the EMS main control unit relies on energy management algorithms to provide bus voltage reference values and output current reference values of any subsystem to the underlying controller (the output current reference value of another subsystem can be calculated by the control algorithm during bus voltage control). Subsequently, the power allocation control algorithm in the underlying controller takes the aforementioned reference values and required feedback data as inputs, and uses the duty cycle of each power electronic switch pulse width modulation (PWM) in the DC/DC converter as the control variable to achieve the underlying feedback control of hybrid energy storage system power allocation.
2.Energy management methods for hybrid energy storage systems
The hybrid energy storage system energy management algorithm takes load demand power and voltage, current, temperature, SOC and other data of LIB and SC subsystems as inputs. Its main function is to provide subsystem output current reference values to the underlying controller (if the bus voltage is controlled, the bus voltage reference value also needs to be provided). The energy management algorithms carried in the hybrid energy storage EMS can be obtained through different hybrid energy storage system energy management methods; Regardless of the complexity of the method, energy management algorithms should have the ability to be applied in real-time online. According to different technical routes, hybrid energy storage system energy management methods can be divided into four categories: experience based, optimization based, working mode recognition based, and machine learning based.
2.1 Energy Management Methods Based on Experience
When the power demand is constant, EMS collects the required information, calculates the reference values of the output power of LIB and SC subsystems, and transmits them to the hybrid energy storage system control unit to achieve power allocation. The most direct way to obtain power allocation reference values is to design a fixed rule based on expert experience, using input parameters such as the required power size and the SOC state of LIB and SC, relying on logic threshold control methods, fuzzy logic control methods, or various filtering methods.
Among them, the mixed storage energy management based on logic threshold control method is a simple, fast, and stable energy management method that compares various real-time input parameters with pre set logic threshold values based on expert experience, and then allocates the output power of LIB and SC according to empirical rules; Energy management based on fuzzy logic control method relies on the principles of fuzzy mathematics, mapping expert experience into a series of energy management rules. This method utilizes the concept of membership function, which can effectively handle fuzzy relationships in hybrid energy storage system power allocation; Based on filtering, energy management starts from smoothing the power output curve of LIB as much as possible, in order to slow down the aging of LIB based on empirical principles. By using methods such as wavelet transform and adaptive filtering, the required power is decomposed into high-frequency and low-frequency parts, which are respectively allocated to SC and LIB.
The traditional logic threshold control method uses fixed logic threshold values for power allocation, and the power allocation results are often unreasonable when there is a significant change in demand power. To solve this problem, the traditional hybrid energy storage system logic threshold power allocation control method has been improved. Under the logic threshold control framework, a fuzzy logic controller designed based on expert experience has been used to replace the fixed logic threshold value. Compared with conventional logic threshold control methods, the improved logic threshold control method has advantages in energy allocation rationality LIB has achieved certain performance improvements in terms of peak output current and energy utilization efficiency. Then, the fuzzy logic method is directly applied for power allocation between the SC and LIB subsystems in hybrid energy storage system. After designing a reasonable membership function to fuzzify the input parameters, fuzzy control rules are defined based on expert experience. Compared with pure battery systems, this greatly reduces the peak output current of LIB and achieves the goal of extending its service life. Reference [61] improves the traditional low-pass filtering method based on fixed cutoff frequency and proposes an adaptive frequency filtering method. Based on this method, an energy management rule is designed, using SC as a low-pass filter to protect LIBs by avoiding frequent charging and discharging. The energy management of hybrid energy storage system is achieved through an adaptive low-pass filter based on a fuzzy logic controller. Compared to the energy management method based on fixed cutoff frequency low-pass filter and the energy management method of pure battery systems, it has a significant improvement in energy utilization efficiency and smooth LIB output power. A self-adaptive hybrid energy storage system power allocation method is proposed, which identifies the power characteristics of load demand through dynamic filtering algorithm, and provides corresponding filtering coefficients based on the fluctuation of power curve. This method can effectively utilize the characteristics of LIB and SC, and delay the aging of LIB.
In the aforementioned energy management methods, based on expert experience, energy management rules generated based on logical threshold methods or fuzzy logic methods have the advantages of simplicity, practicality, high reliability, and good real-time performance. The application of fuzzy logic methods avoids the problem of fixed logical threshold values leading to unreasonable power allocation in certain situations. Starting from the empirical principle of reducing the output power fluctuation of LIB, a filtering based method can achieve the goal of smoothing the LIB output power curve by allocating the high-frequency components of the required power to SC; This type of method can also be combined with fuzzy logic methods to improve the flexibility and rationality of energy management rules.
Although experiential energy management rules are simple, reliable, and highly practical, their optimality is difficult to guarantee due to their determination based on expert knowledge. In addition, scholars generally evaluate the effectiveness of energy management rules obtained through experiential methods from the perspective of whether the rule correctly expresses the input expert experience and whether it conforms to common sense, or choose another simpler empirical rule for qualitative comparison, lacking sufficient quantitative analysis in the effectiveness evaluation of the method.
2.2 Energy management methods based on optimization
The energy management of hybrid energy storage system is essentially to find the optimal power allocation method between LIB and SC under certain conditions, which is easily expressed as a single or multi-objective optimization problem. In power allocation optimization, the optimization objectives are often to minimize hybrid energy storage system energy loss, minimize hybrid energy storage system acquisition and operation costs, and maximize hybrid energy storage system lifespan. The performance constraints of hybrid energy storage system components and load power demand constraints are used as constraints.
According to the need to solve the optimal power allocation problem in real-time during hybrid energy storage system operation, optimization based energy management methods can be divided into two categories: offline optimization based energy management methods and model predictive control based energy management methods.
2.2.1 Energy management method based on offline optimization
Due to the difficulty in ensuring the optimality of experiential energy management rules, researchers hope to design hybrid energy storage system energy management rules based on the solution results of the optimal power allocation problem, in order to reduce dependence on experience and improve the optimality and effectiveness of energy management strategies. Therefore, unlike experiential methods that determine energy management rules based on expert knowledge, offline optimization based energy management methods rely on historical operating data to optimize and solve power allocation problems, and then design energy management rules based on the optimal power allocation scheme obtained from the solution. The energy management framework based on offline optimization is shown in Figure 4.
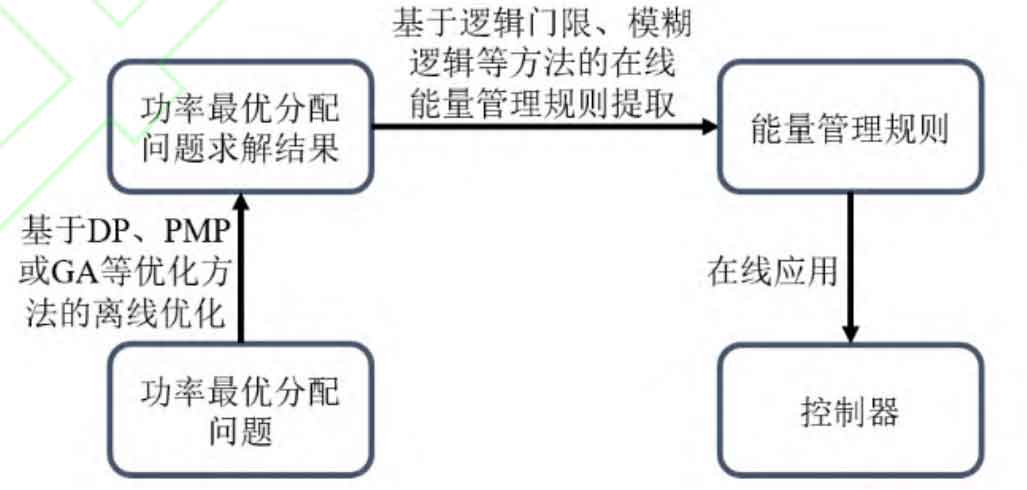
With the continuous development of optimization theory and algorithms, many methods can be used to optimize power allocation. The commonly used methods for solving such optimization problems are dynamic programming (DP), also known as deterministic dynamic programming (DDP), stochastic dynamic programming (SDP) Pontryagin minimum principle (PMP) [72] and various intelligent algorithms such as particle swarm optimization (PSO) and genetic algorithm (GA). These methods have high computational overhead and are difficult to apply as online methods in practice. Therefore, after obtaining the solution set of the optimal power allocation problem, it is generally necessary to extract the optimal power allocation rules that can be applied online through logical threshold or fuzzy logic methods, or discretize the variables in the optimization results. EMS achieves online power allocation through table lookup and interpolation operations. Using DP and PMP methods to solve the hybrid energy storage system power optimal allocation problem, and then evaluating and improving existing energy management rules based on the solution results. After obtaining the global optimal solution of the optimal power allocation control problem through the DP method, the fuzzy logic method is used to generate energy management rules to reduce the influence of human factors. However, the design and deblurring of membership functions still rely on the experience of designers. A pseudo spectral method is proposed by combining spectral method and Gaussian integral method to solve the optimal power allocation problem of hybrid energy storage system, which improves the efficiency of solving the optimal power allocation problem while ensuring the optimality of the solution. The logical threshold method is used to extract energy management rules from the optimal solution.
The energy management method based on optimization starts from the optimization results of power allocation to design rules, avoiding the problem of possible omissions in expert experience; In terms of evaluating the effectiveness of energy management rules, online energy management rules extracted from optimization results through methods such as logical thresholds or fuzzy logic can use the performance of the optimal power allocation results as a comparison benchmark, enabling quantitative rather than qualitative comparative analysis in the past. For known operating conditions, the global optimal solution obtained through precise optimization algorithms such as DP can not only be used to extract online energy management rules, but also use the performance parameters of the global optimal solution in reducing system power loss and slowing down LIB degradation as the upper limit of the energy management method’s efficiency under corresponding operating conditions. The performance of the new method can be analyzed by comparing it with various newly proposed online energy management methods.
2.2.2 Energy management method based on model predictive control
The power allocation optimization in offline optimization based energy management methods relies on historical operating data. When the randomness of future operating conditions is strong and there is a significant difference from historical operating conditions, the performance of energy management using such methods is often poor. To address this issue, some scholars have attempted to apply the Model Predictive Control (MPC) framework to the energy management of hybrid energy storage system. By conducting multi-step prediction with load power demand as a characteristic parameter under the MPC framework, real-time optimization control of hybrid energy storage system power allocation is achieved.
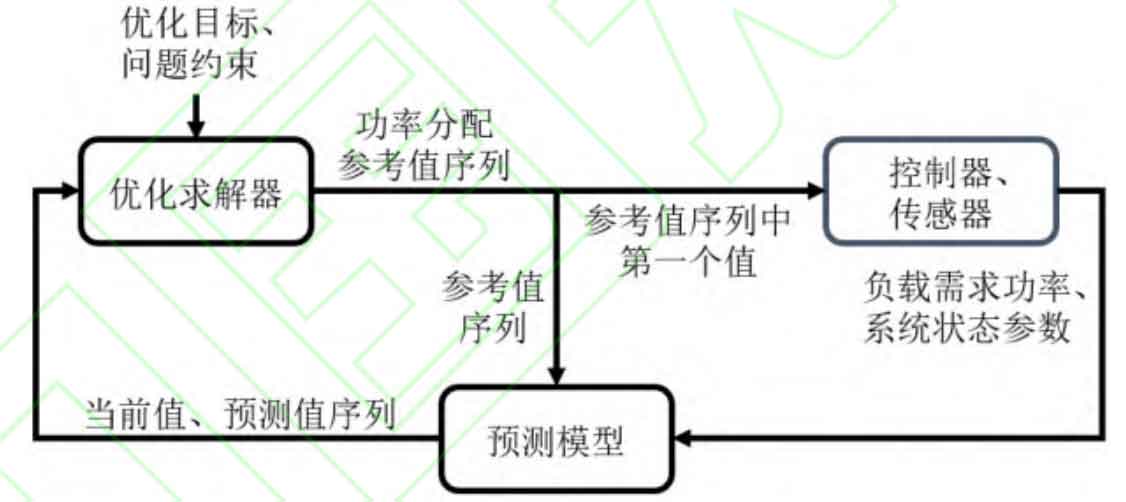
Model predictive control, also known as rolling horizon control (RHC), whose current control action is obtained at each sampling moment by solving a finite time domain open-loop optimal control problem; The MPC method takes the current state as the initial state of the optimal control problem and only executes the first control action in the obtained optimal control sequence. For hybrid energy storage system, the energy management framework based on MPC is shown in Figure 5, where the current and predicted value sequences refer to the SOC, voltage, current, and other parameters of LIB and SC within the current and predicted intervals. The power allocation reference value sequence is the sequence composed of all the power allocation reference values obtained within the control interval; MPC based energy management typically includes the following steps:
1) Predict the operating characteristics (such as the speed of electric vehicles) or demand power of the load for a certain period of time in the future (prediction interval), and use various optimization methods to solve the optimal power allocation problem within the prediction interval in real-time based on the prediction results;
2) Take the first value in the obtained power allocation reference value sequence (control sequence) as the output and transfer it to the power allocation controller to execute power allocation control;
3) Update the current load demand power and the status parameters of LIB and SC at the next moment, and then execute step 1 again.
The performance of energy management based on MPC largely depends on the prediction accuracy of future power demand. Therefore, it is crucial to choose appropriate prediction methods and optimization algorithms within the MPC framework. In energy management based on MPC, prediction of future operating conditions or required power can be achieved using demand torque exponential decreasing model, autoregressive model, Markov chain (MC) model, and neural networks (NN) model. The demand torque exponential decreasing model and autoregressive model methods have low computational complexity, but their accuracy is not high. In recent years, the commonly used prediction methods in energy management systems based on MPC are based on MC and NN methods.
MC prediction models can be divided into first-order MC models, second-order MC models, and higher-order MC models, with increasing computational costs and prediction accuracy. In the case of a small amount of data, the MC model has high computational efficiency and good prediction accuracy; However, the performance of the MC method is poor when predicting based on a large amount of data. At this point, it is possible to consider using the NN method for predicting the required parameters: using deep neural networks (DNN) for prediction, and proving that DNN is more suitable for parameter prediction compared to BP (back propagation) neural networks; A MPC method based on long short term memory (LSTM) speed prediction module was proposed and its effectiveness in hybrid energy storage system energy management was verified.
The control optimization problem in the MPC framework can be solved using optimization methods such as PMP algorithm, DP algorithm, nonlinear programming algorithm, or quadratic programming algorithm. In practical applications, the selection of optimization algorithms needs to consider computational complexity and problem characteristics. For example, considering the real-time feasibility of MPC based on using DP to solve optimization problems within the framework, the DP calculation module is deployed in the cloud, and online energy management is achieved through timed interaction with the onboard MPC module. Overall, the hybrid energy storage system energy management method based on MPC can achieve better results in energy utilization and hybrid storage system lifespan, but its computational cost is also relatively high; When applying online, it is necessary to balance the accuracy and real-time performance of energy management to achieve the best practical performance.
2.3 Energy management method based on operating mode recognition
Similar to the offline optimization based energy management method mentioned earlier, the energy management method based on condition pattern recognition also requires the design of energy management strategies based on historical condition data. The difference lies in that the method based on offline optimization obtains the optimal power allocation scheme for all known historical operating conditions data, and extracts power allocation rules based on this solution result; The method based on working condition pattern recognition divides historical working condition data into multiple different working condition patterns, obtains the corresponding optimal power allocation rules for each working condition pattern in advance, and then identifies the working condition pattern that the current working condition belongs to during hybrid energy storage system operation, applies the corresponding optimal power allocation rules, and responds to the randomness of actual working conditions by regularly re identifying the current working condition. The energy management framework based on operating mode recognition is shown in Figure 6.
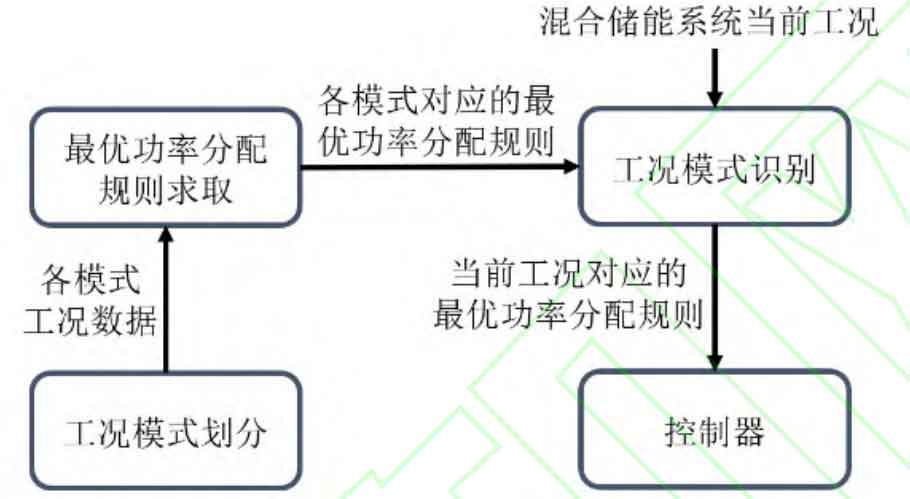
Taking the application of hybrid energy storage system in new energy vehicles as an example, relevant energy management research is often based on standard operating conditions such as the Chinese bus driving cycle (CBDC) or the New European driving cycle (NEDC). By applying the DP method described in 2.2.1, the global optimal power allocation rule under a certain standard operating condition can be obtained. The initial energy management method based on condition pattern recognition directly identified various standard cycle conditions as different operating modes. The division of operating modes in this way is too rough, and the actual driving conditions are often difficult to have sufficient similarity with various standard operating conditions. Therefore, the energy management method of directly using standard cycle operating conditions as operating modes is not effective in practical applications. In response to this issue, scholars consider dividing the standard cycle operating conditions into multiple “microcycles” and obtaining optimal power allocation rules based on these “microcycles”. Assuming that two adjacent microcycles have similar operating characteristics, appropriate power allocation rules are applied to online energy management through real-time “microcycle” pattern recognition.
The “microcycle” can be separated by a point with a power demand of 0 in the standard cycle operating conditions, or it can be directly divided by time length (such as every 60 seconds or 100 seconds), or a maximum duration of the “microcycle” can be set. If the “microcycle” to be divided is lower than the maximum duration, the point with a power demand of 0 in the standard cycle operating conditions can be used as the separation point, When the “microcirculation” to be divided exceeds the maximum duration, the “microcirculation” is forcibly divided according to the maximum duration. After the division of the “microcycle” is completed, the working mode recognition framework is combined with optimization based methods to obtain the optimal control rules for the divided driving “microcycle” working conditions through DP. Then, with the average and maximum driving speeds in the “microcycle” working conditions as inputs, the real-time working conditions are matched with the pre divided “microcycle” through fuzzy logic method, and the corresponding optimal energy management rules are applied. Reference [12] has improved the aforementioned method by using the energy demand and maximum power demand of the operating conditions as inputs for fuzzy logic pattern recognition, matching real-time operating conditions with the “microcycle”, and then applying corresponding energy management rules; This rule is also based on the divided “microcycle” working condition data, which is optimized and calculated using DP in advance. The fuzzy logic method is also used to identify operating modes in real-time, but the difference lies in its use of particle swarm optimization to optimize hybrid energy storage system power allocation under “microcycle” operating conditions. Using real-time speed and power demand in driving conditions for mode recognition, the energy management rules corresponding to each “microcycle” are obtained through NN fitting of the optimal power allocation curve parameters. Under the mode recognition framework, the adaptive wavelet transform method is used for power allocation, and the decomposition level of the wavelet transform is dynamically adjusted based on the identification results of the “microcycle”, And combined with fuzzy logic control to maintain the SOC of SC within the preset range.
Most energy management methods based on condition pattern recognition combine optimization based methods to obtain the optimal energy management rules for a specific cycle condition. Therefore, the performance of energy management methods based on condition pattern recognition in practical applications largely depends on the recognition accuracy. In recent years, with the continuous improvement of researchers, the overall operating mode recognition method can achieve better energy management efficiency with lower computational costs. However, due to the fact that the pattern recognition module usually recognizes the current operating mode based on historical information and assumes that the current operating characteristics will not suddenly change, it is not possible to completely avoid misidentification and may not achieve optimal power allocation in some cases.
2.4 Energy Management Method Based on Machine Learning
Machine learning methods are an important future development direction in the field of system control. In recent years, machine learning related technologies represented by NN methods and reinforcement learning (RL) methods have developed rapidly and have been applied in many aspects such as robot control, natural language recognition, and autonomous driving. Considering the wide applicability of machine learning methods, their excellent performance in handling complex nonlinear problems, and their excellent real-time performance after training, many scholars have attempted to apply them to the field of hybrid energy storage system energy management.
NN is a mathematical model that mimics the structure and function of biological neural networks (the central nervous system of animals, especially the brain). It can simulate the interactive reactions of biological neural systems to real-world objects, and its structural diagram is shown in Figure 7. When applied, NN requires a large number of training datasets to train it, which is essentially an inductive learning. During the repeated learning process of a large number of training instances, the adaptive algorithm within the NN model continuously modifies the weights of connections between neurons and gradually converges the weight distribution to a stable range.
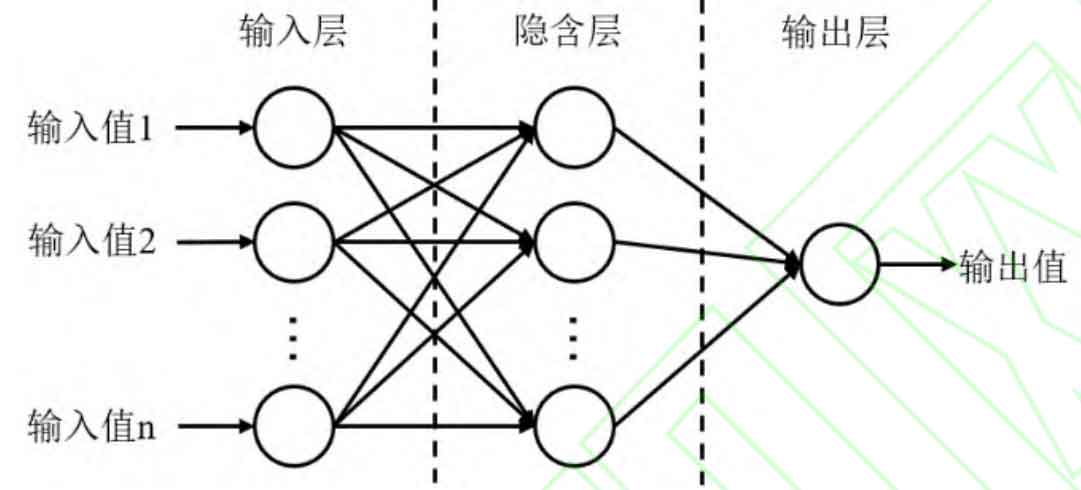
RL can not only utilize existing data, but also obtain feedback (rewards) through continuous interaction with the environment, and iteratively update the existing model with new feedback data to obtain the global optimal behavior strategy, as shown in Figure 8. For systems with unknown system structures and parameters, RL can control them by observing and analyzing their behavior, and make optimal decisions through gradual learning or trial and error.
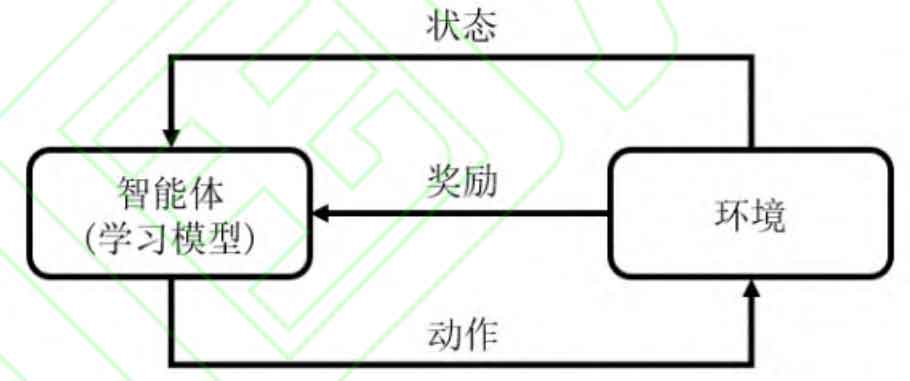
By using the hybrid energy storage system power allocation data obtained from the DP algorithm, a NN based hybrid energy storage system controller was trained, and real-time application and verification of optimized data were achieved. Replacing the rules of the PI feedback controller with NN obtained through data training improved the energy utilization efficiency of hybrid energy storage system by 3.3%. A power allocation controller based on NN has been designed, achieving lower error and higher stability than PI controller, effectively improving hybrid energy storage system efficiency and extending its service life. The NN is trained offline using a dataset obtained based on wavelet transform, and the high-frequency components in the load power demand are allocated to the SC based on the trained NN. A low voltage DC microgrid hybrid energy storage system control strategy based on NN has been proposed, which can quickly track the high-frequency components in power demand, maintain the SOC of the battery and SC within the preset range, and stabilize the bus voltage. Reference improved the low voltage DC microgrid hybrid energy storage system control strategy based on NN, using the bat search algorithm to solve the feedback gain of the controller offline, and trained the NN for online application based on the obtained control signal dataset to achieve fast online calculation of feedback gain. To improve the transient performance of hybrid energy storage system power allocation, NN was used for system control, and significant results were achieved. For the hybrid energy storage system EMS based on NN, the number and quality of training sets and the applicability of the established NN model have a direct impact on the final energy management performance. These factors need to be comprehensively considered when establishing and training NN.
Applying RL to real-time energy management of hybrid energy storage system, the effectiveness of RL method in hybrid energy storage system energy management was verified by comparing the simulation results obtained by RL based method and DP based method. Reference is based on the RL method considering forgetting factors, which simultaneously considers the effects of LIB lifetime and temperature changes on power allocation in hybrid energy storage system energy management. Experiments have shown that the RL based method can reduce energy loss by 16.8% compared to rule-based control strategies. Under the deep reinforcement learning (DRL) framework that combines deep learning (DL) and RL, the soft actor critical (SAC) algorithm is applied to the energy management of hybrid energy storage system. The knowledge extracted from the global optimal energy management scheme based on DP is used to improve the EMS performance of SAC based hybrid energy storage systems, Ultimately, the performance gap between this EMS and the global optimal EMS based on DP was reduced to 5.19%. Based on the multi-agent DRL method, a collaborative control strategy between wind turbines and hybrid energy storage system is proposed by combining hybrid energy storage system energy management with wind turbine rotor kinetic energy and pitch control; Simulation experiments have shown that this strategy can smooth the output power of wind power generation, extend the service life of energy storage components, and reduce the wear of wind turbines. At present, the hybrid energy storage system EMS based on RL or DRL still faces problems such as difficulty in parameter tuning and long training time. When applying RL and DRL methods for hybrid energy storage system energy management, it is necessary to focus on the convergence speed, optimality, and real-time performance of the methods.
In addition, machine learning methods are often applied as an effective parameter fitting and prediction method in other types of hybrid energy storage system energy management framework based on experience, operating mode recognition, etc. In these applications, NN is not used to directly output reference values for hybrid energy storage system power allocation, so this article does not classify these application methods as machine learning based energy management methods.
The machine learning based hybrid energy storage system energy management method can achieve performance similar to the optimal energy management rules obtained by DP in reducing hybrid energy storage system power loss, extending hybrid energy storage system service life, and improving hybrid energy storage system operation economy; Compared to the aforementioned methods based on operating mode recognition or optimization, machine learning based methods have better real-time performance and robustness. However, to ensure the efficiency of energy management, machine learning based hybrid energy storage system energy management methods often require a long training time and a large amount of high-quality training data during learning, which to some extent limits the application of such methods.
2.5 Summary of Energy Management Methods for Hybrid Energy Storage Systems
The four major types of energy management methods based on experience, optimization, working mode recognition, and machine learning can be considered as mainstream hybrid energy storage system energy management methods that each represent a stage. In terms of research depth, they roughly follow a gradually deepening and progressive relationship. However, all four types of methods still have irreplaceable advantages in certain aspects, and also have unavoidable disadvantages. Here, the load conditions that hybrid energy storage system needs to deal with are first divided into two categories: regular operating conditions that can be obtained through historical operating data in the future, and random operating conditions that exhibit random fluctuation characteristics and cannot be accurately obtained through historical operating data. The performance characteristics of various energy management methods are summarized in Table 3.
Energy management methods | Regular working condition management efficiency | Randomness working condition management efficiency | Robustness | Offline computing complexity | Online computing complexity |
Based on logic threshold/fuzzy logic | Moderate | Poor | Moderate | Lower | Very low |
Based on filtering | Moderate | Poor | Moderate | Lower | Very low |
Based on offline optimization | Good | Moderate | Moderate | Higher | Very low |
Model based predictive control | Better | Good | Good | Very low | higher |
Based on operating mode recognition | Better | Better | Better | Moderate | Moderate |
Based on machine learning | Better | Better | Good | Very high | Lower |
In Table 3, the performance characteristics of various energy management methods are evaluated from five aspects: management efficiency, robustness, offline computing complexity, and online computing complexity under regular and random load conditions. The evaluation of management efficiency and robustness is divided into four levels from good to bad: good, good, medium, and poor. The evaluation of computational complexity is divided into very low, low, medium, and high from low complexity to high complexity There are five high levels. Among them, management efficiency represents the performance of energy management methods in improving the efficiency of system energy utilization and extending the overall lifespan of the system; Robustness is evaluated based on whether the management effectiveness of energy management methods is sufficiently stable when dealing with sudden abnormal operating conditions; The complexity of offline and online computing is evaluated separately for the computational costs of energy management methods in both offline and online real-time computing.
The research on hybrid energy storage system energy management has been committed to achieving good management efficiency and robustness even under strong randomness and large fluctuations in load conditions. From experience based, optimization based, to condition pattern recognition based, and machine learning based methods, their improvement in management efficiency and robustness compared to other methods in dealing with stochastic conditions often comes at the cost of increasing offline or online computational complexity. For regular operating conditions, the simplest energy management methods based on experience and offline optimization can effectively extend the overall lifespan of hybrid energy storage system; Especially based on offline optimization methods, which rely on the global optimal solution of the load demand power optimal allocation problem to obtain energy management rules, they can achieve better energy management efficiency when dealing with regular load conditions compared to energy management methods based on model predictive control, condition pattern recognition, and machine learning, which have higher offline or online computational complexity and more complex method processes. Therefore, for hybrid energy storage system facing regular operating conditions, using offline optimization methods can achieve good energy management efficiency, and generally, more complex methods are not necessary; For hybrid energy storage system facing random operating conditions, the selection of energy management methods needs to comprehensively balance the practical application requirements of management efficiency, robustness, and computational complexity, as well as the degree of random fluctuations in hybrid energy storage system load conditions.
3. Future research prospects for energy management in hybrid energy storage systems
Developing an energy management method with good management efficiency, strong robustness, and minimal computational complexity for hybrid energy storage system targeting stochastic loads, especially strong stochastic loads represented by electric vehicles, is currently and will be the main goal of hybrid energy storage system research for a considerable period of time in the future. To achieve this goal, there are still three urgent issues to be addressed at this stage:
Firstly, improve the prediction accuracy of future operating conditions for random loads. For random loads, the smaller the uncertainty of their future operating conditions, the more likely they are to achieve higher management efficiency and robustness at lower computational costs. By collecting more comprehensive data related to load conditions, introducing advanced prediction models and algorithms, and further considering the different operating styles of load operators in the prediction, it is possible to make more accurate predictions of the future load conditions that hybrid energy storage system needs to cope with, reducing the uncertainty of future load conditions as much as possible, and thereby improving the efficiency and robustness of hybrid energy storage system energy management. So, how to organically combine effective prediction models with existing energy management methods to achieve the goal of improving the efficiency and robustness of hybrid energy storage system energy management while meeting practical requirements in computational complexity is an urgent problem that needs to be solved.
Secondly, establish a more accurate hybrid energy storage system model. For energy management methods based on optimization and working mode recognition that rely on hybrid energy storage system dynamic response models and aging models, the accuracy of the models they rely on will directly affect the actual efficiency of energy management. At present, relevant energy management methods have the problem of selecting models that are too simple and fail to closely fit the actual working conditions of hybrid energy storage system, often making it difficult to accurately reflect the voltage and current response and aging of hybrid energy storage system under special working conditions such as low temperature or high rate discharge. Therefore, how to fully consider the actual working conditions and operating environment of hybrid energy storage system, and accurately model the hybrid energy storage system model as the foundation of energy management methods, is an urgent problem that needs to be solved at present.
Thirdly, further enhance the real-time performance of energy management methods through cloud collaboration. During the operation of hybrid energy storage system, real-time power allocation control is required, which places high demands on the real-time performance of energy management methods when applied online. In existing research, energy management methods with high online computational complexity, such as model predictive control and operating mode recognition, often find it difficult to have sufficient real-time performance in practical use; Failure to provide power allocation reference values to the controller in a timely manner, or failure to update energy management strategies in a timely manner, will directly affect the management efficiency and robustness of these energy management methods. At present, scholars have made preliminary explorations in combining model predictive control or machine learning based energy management methods with cloud computing. However, there is still significant room for exploration in further improving the real-time performance and robustness of energy management methods through cloud collaboration.
In addition, most of the existing research on hybrid energy storage system energy management focuses on electric vehicle hybrid energy storage system, while a small portion focuses on hybrid storage power sources for other transportation vehicles such as light rail and ships, as well as hybrid energy storage system in other application scenarios such as power grid energy storage. At a time when advanced achievements in energy management of electric vehicle hybrid energy storage system are constantly emerging, research on energy management methods for hybrid energy storage system designed for other types of load conditions and operating environmental conditions still needs to be further deepened.
4. Conclusion
Firstly, a brief introduction was given to the controlled and uncontrolled topologies of the LIB/SC hybrid energy storage system. The connection methods and main characteristics of the active, semi-active, and passive topologies were analyzed, and the functional architecture and power allocation control flow of the LIB/SC hybrid energy storage system EMS were summarized; Then, four major types of energy management methods for LIB/SC hybrid energy storage systems based on experience, optimization, operating mode recognition, and machine learning were emphasized. The specific processes and performance characteristics of each type of energy management method were classified, detailed, and compared and analyzed; Finally, the performance characteristics of existing energy management methods in terms of management efficiency, robustness, and computational complexity were summarized, and the future research directions and development trends of energy management methods for LIB/SC hybrid energy storage systems were prospected.